Ann-Kristin Becker’s Bayesian network assesses the risk of developing the liver disease more accurately than common clinical scores
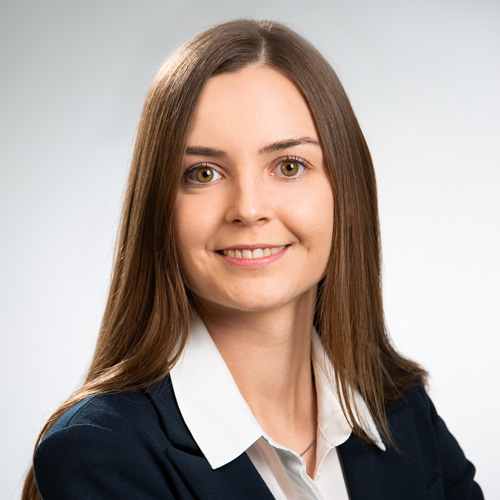
Making sense of the jungle of systems biological data: Ann-Kristin Becker, a member of the LiSyM research network, has determined which of the 500 variables from a large amount of health data contribute to nonalcoholic fatty liver disease, or NAFLD, and to what degree. This is a difficult task, because nature – humans included – follows certain rules, but never one hundred percent. Nevertheless, she was able to single out all relevant relationships using her Bayesian network (BN). The model created by Becker, a bioinformatics PhD student in Professor Dr. Lars Kaderalis’s research lab at the University of Greifswald, presents the relationships in a clear and understandable way. Her BN also predicts the NAFLD risk more precisely than common procedures. Becker is currently feeding gene expression data into the BN. This means that a simple diagnostic test for NAFLD, which researchers have long been working toward, may finally be within reach.
“Learning the BN structure is very complex if there are large sets of data, but it’s worth it!” says Becker. In initial tests, her network of relationships between health variables and NAFLD calculated the likelihood of developing the disease more accurately than common clinical scores.1 “Furthermore, with the network I can investigate what impact different combinations of variables have on this risk,” she explains. Her BN is able to estimate how high the NAFLD risk is, for example, for a 56-year-old man. Every additional piece of information refines the result. For instance, the risk for this 56-year-old man increases or decreases if he weighs more or less, respectively. The BN therefore shows if, and if so to what degree variables, individual and in combination, affect the probability of developing NAFLD.
Simple NAFLD diagnostic tests are not available
Becker is currently working on a subproject for her BN. “The goal is to include the gene expression data from patients’ blood together with the epidemiological data,” she says. Reliable and measurable blood parameters for NAFLD could be the key to making simple diagnostic tests possible. Experts have been seeking such a solution for a long time. NAFLD affects 20–30 percent of the population in Europe, meaning the disease, which can lead to cirrhosis and cancer of the liver, is widespread. And yet NAFLD often goes undetected for many years and is often only discovered at a late stage. One reason for this is that doctors do not have any simple tools to diagnose it.
4,000 anonymized data sets form the foundation
Becker’s BN is based on data from the Study of Health in Pomerania, or SHIP, for which the Institute of Community Medicine of the Faculty of Medicine at the University of Greifswald has been collecting and documenting the general epidemiological health data of several thousand randomly chosen participants since 1997. Becker used 4,000 anonymized data sets from this study to learn, train, and test her BN. This wealth of data consists of roughly 500 different variables – not only many health readings, but also information provided by patients concerning their lifestyle, how they feel, and other similar data. Simply put, the BN gauges the probability of relationships between the variables in these data. For example, symptom X is found in 30 percent of the cases of disease Y in the data. As Becker says, “If there are lots of relevant data, I can deduce for any patient with disease Y that there is a probability of 30 percent that that patient with will have symptom X.” Thus, calculations linking more than two variables provide clues about dependencies and how strong these may be. To do this, Bayesian networks combine basic statistical methods with machine learning and artificial intelligence.
BNs show whether each variable has a direct or indirect effect
“BNs primarily model probability distributions,” explains Becker. Depending on how variables are weighted and their specifications, there are very many possibilities for how 500 variables could theoretically be related. This is why there are so many different BN structures in the beginning and why a selection and optimization is then conducted using a kind of plausibility score. This disqualifies models that reveal contradictions, for example. Finally, the BN attains an optimum state once the best structure is found using a hill climbing algorithm. “A major advantage of BNs is that they are directed,” says Becker. They never contain connections which backtrack or are circular. Relationships between variables always follow in the one direction, and never form a circle, not even over several nodes. As a result, it is clear which variables a particular one is directly connected to, and which variables it is indirectly connected to via one, two, three, or more variables.
For more details, clusters of variables can be disbanded
Becker needed to modify the standard BN described above for her purposes, however. Because the mass of data and variables were so large, it would have taken a long time to compute the model and would have resulted in a BN that was too big and too chaotic. “That’s why I clustered the variables,” Becker explains, adding: “I took the ones that were very similar in content and basically condensed them into one variable.” In this way, she was able to create a network that can be clearly understood and shows the most important relationships. “The key was to not make the clusters too broad,” she says. She also made certain that no information was missing. Therefore, if the details of a cluster are of interest, all she has to do is zoom in on the relevant cluster of variables in the BN and disband them again.
Predictions are more accurate than established clinical scores
“None of the relationships were a big surprise,” she says. There are many useful clinical variables in the SHIP study that have been thoroughly scrutinized for their role in the spread of diseases. However, Becker’s BN is a dynamic mathematical model that does more than just reveal relationships. It can also run hypotheses, estimate the effect of single or combined variables for NAFLD, and calculate the probability of developing this disease. When Becker checked the quality of these outcomes against a subset of the SHIP data that she had previously excluded during the development of her BN, her BN’s predictions were more precise than the established clinical scores.1
Gene expression data should improve predictions
“Gene expression data should improve predictions even more,” says Becker. To date, knowledge of how gene expression changes at the onset and in the course of NAFLD is almost entirely based on liver cells. “Initial results indicate that primarily the iron metabolism changes in the beginning,” she explains. Later, immune and infection responses can also be detected in the blood. The sample material needed to examine cells is taken from a liver biopsy in which a small amount of tissue is surgically removed. This procedure is still necessary to be able to diagnose NAFLD with certainty. A biopsy is performed when patients express typical symptoms or if blood test results provide strong evidence of NAFLD. Although the risks involved are estimated to be low, a biopsy on a patient who otherwise seems healthy to confirm a vague suspicion cannot be justified.
A blood test for everyday clinical use is getting closer…
Becker points out that, in contrast, most people do not mind having a blood test. “It would be easy to integrate a blood test to detect NAFLD in everyday clinical practice,” she says. Gene expression data was also obtained from blood samples of some participants in the SHIP project. “Unfortunately, the research into identifying NAFLD-specific signatures in blood is really only in the beginning phase,” Becker says. Nevertheless, she plans to integrate all the data SHIP has to offer into her epidemiological BN. This will increase the number of variables. However, it will also mean there are fewer data available to train the BN. “I’ll have to do a little fine-tuning to get a robust network,” she says. Ultimately, models that include gene expression data in addition to basic epidemiological data can usually predict diseases better.
We need more collaboration between computer science and clinical practice!
A complete BN for NAFLD could thus be the foundation for diagnostic blood tests in the near future. Before Becker takes up a new position in 2021, she wants to make as much progress on her BN as possible – perhaps it will even be able to identify a few additional metabolic pathways that play a role in the progression of NAFLD someday. Until then, Becker says she would be elated if her network could help to improve the situation of NAFLD patients in any way possible. As to the clinical side, she is happy to leave that to others, she says, “It would be great if my BN could help to promote the exchange of ideas between clinical practice and computer science.” Ultimately, she would like to see the two fields collaborate more, adding: “I think it’s important for people to be less afraid of statistical models.”
1 : Fatty Liver Index, Hepatic Steatosis Index, NAFLD ridge score